The Role of AI in the Finance Industry
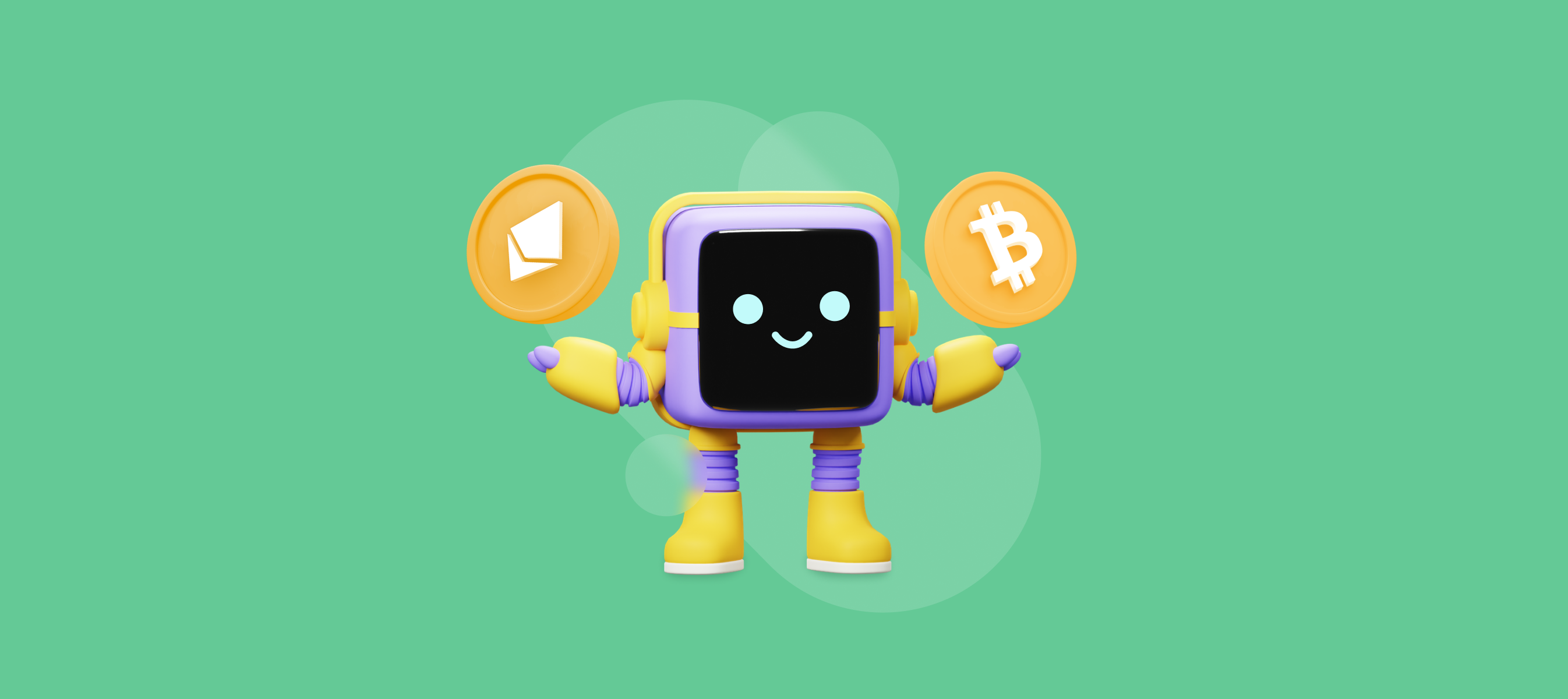
This blog post will cover:
- Practical implications and applications of ML
- How can you leverage deep learning?
- To what extent can AI identify fraud in the market?
- Global corporations using AI to fight crime
- Benefits and drawbacks of AI in finance
- Conclusion
ML is an element of AI, whose algorithms are able to analyze huge volumes of financial information to identify potential anomalies, which could mean someone is trying to perpetrate fraud. These models use historical data to identify the risk of fraud in real time, at least in theory.
What actually happens, though, is that financial institutions can sort clients into profiles using ML based on their previous financial operations. These profiles provide an updated and reliable picture of a client’s activity and facilitate accurate forecasts.
A client’s account might be profiled as “travels to New York once every few months” or “eats out on Tuesdays.” The profile is updated after each transaction. AI determines whether an operation is “normal” or deviates from the norm and justifies an alert.
Practical implications and applications of ML
We use machine learning to create sophisticated commercial algorithms, which use data to make investment decisions. They can process market trends, sentiment, historical data, and other important information to attain trading signals or improve fiscal portfolios.
- Risk evaluation and controlML-based models can assist in evaluating and limiting diverse types of financial risk. They can forecast how likely someone is to pay a loan back, predict how likely an insurance claim is, or assess market risk based on previous records.
- Evaluating creditworthinessML algorithms can be deployed to evaluate how creditworthy a client might be by assessing past information like debt and payment history. Lenders can train models on financial records to prognose an individual's credit risk more accurately. They can decide whether to provide a loan and if so, on what terms.
- Segmenting clientsML techniques can assist banks in sorting their clients and making targeted proposals. They can tailor services and products by analyzing data like client behavior and transaction records.
Natural language processing is also actively used in the financial sector. NLP, a component of machine learning, can be used to evaluate text, such as media reports, social network information, and earnings data. Banks can leverage NLP mechanisms to gain insight and understand market sentiment as well as obtain more knowledge of market trends.
How can you leverage deep learning?
RNNs, LSTMs, and other deep learning templates predict share prices, exchange rates, and other financial developments based on historical data. This enables them to grasp complicated patterns and dependencies in the information analyzed, allowing for more precise estimates.
RNNs and CNNs are leveraged to analyze market attitudes based on large text information volumes, including media reports. Banks can leverage the breakdown to guess what impact news events might have on the market.
To what extent can AI identify fraud in the market?
The average damage from cybersecurity breaches reported is 7% of company revenue. Losses from digital fraud are expected to reach almost $350 billion globally in the next four years.
AI studies past data and comes up with “risk rules,” which users apply to flag specific actions, such as suspicious login attempts. Not all ML devices are made equal, however. It’s key to be aware of the distinction between whitebox and blackbox ML.
Blackbox ML is the more rudimentary option. Decisions are automatic and non-transparent. If you don’t need in-depth insight into risk analysis, this system will work for you.
Whitebox learning systems, on the other hand, explain why they suggested a risk rule clearly. Those tasked with fraud management will understand the source of risk more easily and adapt their prevention strategy, if needed.
Global corporations using AI to fight crime
Corporations like Mastercard, PayPal, and JPMorgan Chase are using AI to fight fraud and reap the rewards.
Mastercard’s Decision Intelligence product uses past data from users’ buying habits to create a baseline and check for unusual behavior.
PayPal leverages ML algorithms and applies risk rules to detect high-risk processes and survey transactions as they occur. Its losses from financial crimes are 0.32% of its revenue, compared to an average of 1.32% for analogous online merchants.
JPMorgan Chase identifies potentially criminal activity by applying a proprietary algorithm. The details of each financial transaction are forwarded to data center computers, which assess them for signs of fraud. Chase has great results on surveys, in terms of being reliable and secure.
Last but not least, U.S. Bancorp uses AI in various office applications. Bancorp is a household name in US banking. It takes advantage of deep learning tools to study important client data and detect potentially malicious entities. Since it began to use AI to stop money laundering, it has become twice as productive according to an Insider Intelligence report.
Benefits and drawbacks of AI in finance
AI tools can process and study huge volumes of data fast and easily, enabling better decision-making. This results in higher precision and efficiency in assessing risk and detecting fraud.
This is a double-edged sword: because AI algorithms process so much data, data privacy faces a growing risk.
Pluses
- 24/7 supportBots and digital assistants provide support around the clock. They can address clients’ questions and help with basic fiscal tasks.
- Better risk controlAI tools can evaluate and control risks more efficiently by considering a large number of data inputs, past tendencies, and market conditions. This allows banks to establish potential risks, create an appropriate risk control approach, and make better decisions about loans, investment products, and managing client portfolios.
- Automation and effectivenessAI tools can carry out financial tasks automatically, including mundane and drudge work ones. This results in more efficiency and helps companies save money. Tasks like entering data, processing documents, and customer service can become automatic.
Minuses
- Discrimination risksAI tools can inadvertently perpetuate data bias because the information used to train them is biased itself. This has the potential to result in discrimination.
- Absence of interpretabilityIt’s hard to grasp the perspective underlying decisions made by deep learning tools. This absence of interpretability can raise questions about being transparent and holding people accountable and compliant.
- System vulnerabilityMechanical failures, adversarial bouts, or unexpected situations not encountered during training can result in mistakes or system vulnerabilities.
Conclusion
Finally, using AI tools compels organizations to make sure they operate in a transparent and fair way. They need to address concerns involving job displacement, algorithmic accountability, and the effect on vulnerable populations.
What awaits us in the near future? Will AI be introduced into the routine work of the financial sector? Time will tell and SimpleSwap will keep an eye on the situation to give you the freshest updates.
If you are interested in AI and ML, you may check out our previous articles: We Asked AI’s “Opinion” About Crypto, AI Revolution in Crypto and AI: A Loyal Friend or the Worst Enemy?